Data science and artificial intelligence (AI) are two phrases that have become popular in academic and industry circles in this age of rapid technological innovation. Even though these phrases are frequently used synonymously, they have a unique yet connected relationship. This article explores the depths of data science and artificial intelligence (AI) to reveal their shared and unique characteristics as well as the complex relationships that unite them.
Knowing Data Science
The broad discipline of data science includes a range of methods, procedures, and algorithms for drawing conclusions and information from both structured and unstructured data. Fundamentally, data science seeks to convert unprocessed data into useful insights that can be used to improve corporate operations across many industries and facilitate well-informed decision-making.
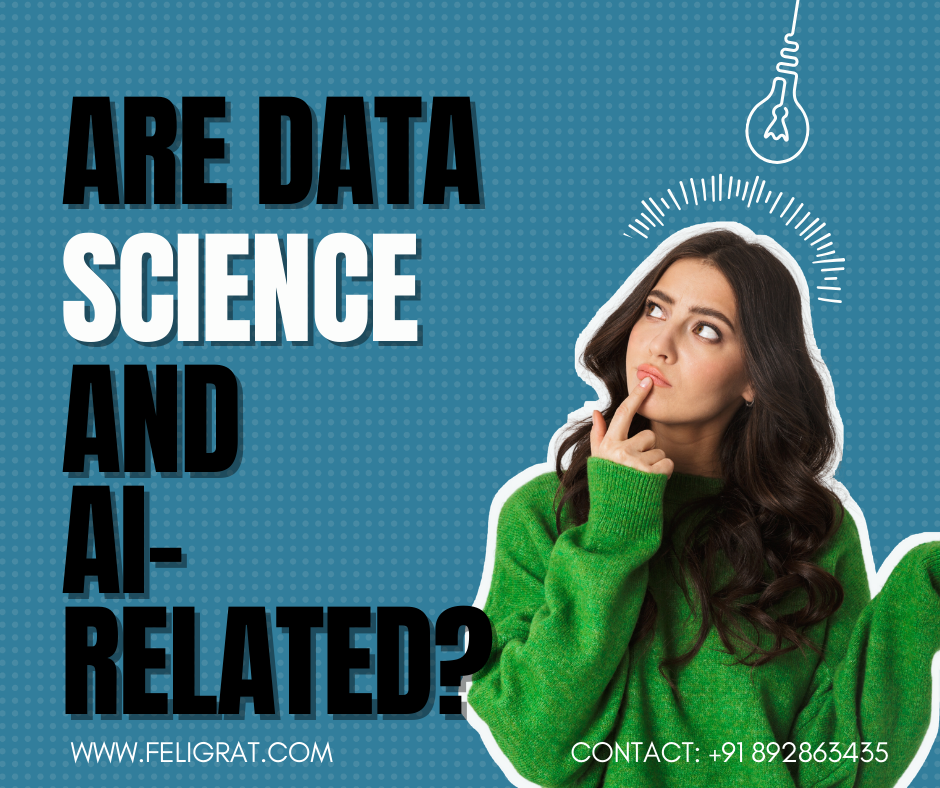
Data science is a methodical approach to data analysis that combines domain expertise, statistical methodologies, machine learning algorithms, and data visualization tools. The ultimate objective of this process is to extract significant patterns and trends from complicated datasets using data collection, preprocessing, exploration, modeling, evaluation, and interpretation.
Interpreting Machine Intelligence:
Conversely, artificial intelligence (AI) is the creation of computer systems that can carry out operations that normally call for human intelligence. Numerous cognitive processes are involved in these tasks, including perception, thinking, learning, problem-solving, and decision-making.
Symbolic AI and machine learning are the two primary subtypes of AI approaches. While machine learning methods allow systems to discover patterns and relationships from data, improving their performance over time, symbolic AI depends on established rules and logic to accomplish tasks.
To learn more about SAP Courses – Click Here
The Point Where AI and Data Science Collide:
Despite being separate disciplines, data science and AI work well together, with one utilizing the other’s advantages to spur innovation and advancement. Because data science provides the raw material — data — that powers AI algorithms and models, it acts as the cornerstone upon which AI grows.
Data Gathering and Preparation:
To guarantee that the data is of high quality and appropriate for AI applications, data scientists must gather, clean, and preprocess the data. Robust and reliable AI models require training with high-quality data.
Feature Engineering:
To aid AI algorithms in their learning process, feature engineering entails the identification, extraction, and transformation of pertinent features from unprocessed data. Data scientists use their imagination and domain expertise to build informative features that improve AI model performance.
Model Training and Evaluation:
Data scientists train artificial intelligence (AI) models using labeled datasets by applying statistical approaches and machine learning algorithms. They then fine-tune the models’ parameters to maximize performance. Strict assessment techniques are used to evaluate AI models’ generalization, accuracy, and dependability.
Explainability and Interpretation of AI Models:
Data scientists are essential in deciphering the inner workings of AI models and elucidating their conclusions to interested parties. This entails evaluating model outputs, spotting key characteristics, and guaranteeing accountability and openness in AI-driven decision-making.
Feligrat’s course offers expert data science instruction for all experience levels, ensuring job stability and respect. Gain real-world project experience and confidently enter the industry.